Social Impact
The implementation and use of algorithms by public entities and private organisations always have some kind of consequences on social life, as they are often based on data about the public and deal with the distribution of public resources and the delivery of public services. If the data are biased or if the algorithms don’t work as intended or are designed to discriminate among people in an unjust way, then the use of such algorithms will have a negative side and harmful effects, and that’s what we mean here by social impact: threat to people’s privacy; unjust discrimination based on gender, race, religion, socioeconomic status or other issue; reproduction of existing inequality; weakening of democratic practices; state surveillance…
Algorithmic Discrimination
A biased algorithm is an algorithm that systematically and unfairly discriminates against certain individuals or groups of individuals in favour of others. A system discriminates unfairly if it denies an opportunity or a good or if it assigns an undesirable outcome to an individual or group of individuals on grounds that are unreasonable or inappropriate’
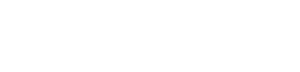
There are different sources of potential bias that can statistically affect algorithm outcomes throughout their life-cycle, but the social impact will be constrained by the socially-given meaning of the resulting discrimination or harmful effect.
It is due to those reasons that we need to keep in mind that, despite their seemingly neutral mathematical nature, an algorithm developed for a concrete service and taking all reasonable steps in its design, may still produce and reproduce biases that unjustly discriminate against women, people of colour, minorities, the poor and other traditionally excluded groups.
The Social Impact of Algorithms
Having processed more than a hundred algorithms of different kinds and aiming to tackle algorithmic bias rigorously and systematically, the Eticas Foundation team has defined the following discrimination taxonomy.
Racial discrimination
This refers to discrimination against individuals or groups on the basis of race, colour, descent, national origin or ethnic or immigrant status. An example would be some recidivism-predicting systems, which have been proven to be inefficient and racially biased against some populations.
Gender discrimination
This is a common violation of human rights based on the gender identify or sexual orientation of an individual. In machine learning, gender discrimination can take the form of employment searching algorithms offering less paid jobs or worst job opportunities to women than men, for instance.
Socioeconomic discrimination
This is the prejudice against individuals based on their income, level of education, professional status and/or social class. An example could be when insurance companies use machine-learning algorithms to mine data –such as shopping history– to label some customers as high-risk to charge them more for their insurance packages.
Religious discrimination
This consists in treating a person or group differently because of the beliefs they hold. As an example, research has shown that some machine-learning algorithms were using words related to the Muslim community to search for misconduct and potentially risky behaviour on social media.
State surveillance
Some algorithmic systems may contribute to practices of surveillance of individuals or groups by state bodies or by private organisations that are not the result of due process, which haven't been properly sanctioned or audited, or which aren't transparent and respectful of people's rights.
Threat to people's privacy
Automated decisions made without human judgment may affect the right of individuals to their own private sphere. This threat is boosted by the large amounts of data processed by algorithmic systems (for example, data from people's social media use), which allows public and private organisations to infer highly sensitive and private information about individuals .
Generating addiction
Some algorithms may contribute to make people addicted to using or relying on particular products or activities in an unhealthy or otherwise harming way. That can happen, for instance, when gaming apps, social media or broadcasting services behave strategically to keep users in the app for as long as possible.
Social polarisation / radicalisation
The implementation of some algorithms may result in the production or distribution of online content that contributes to push individuals or groups towards extreme attitudes or behaviour. For example, algorithms used in social media may promote extreme and even violent content because it gets more clicks.
Manipulation / behavioural change
In some cases, algorithms may purposely or inadvertently contribute to modify people's thinking, beliefs or way of behaving or acting without their awareness or in an unhealthy or otherwise harming way. That can be the case when algorithms produce highly targeted and personalised propaganda or advertising that manipulate the way people normally behave.
Disseminating misinformation
The use of algorithms may result in the production or distribution of online content that's purposely untrue, wrong, partial or that in other way contributes to make people think or believe something that's not true. That has been the case, for instance, regarding the climate crisis or the use of vaccines, about which there is scientific consensus.
Interested in our work?
You can collaborate with the project by sharing with us algorithms that are being implemented around you or by using the information in this directory to foster changes in your community.