Auditing algorithms
Often, algorithmic systems are what’s known as “black boxes” because it’s not publicly known how they work: we don’t know what goes on inside the “box”. In the case of machine-learning algorithms, the systems may become black boxes even to the people who designed them, because the algorithms can rewrite their own rules. When such algorithms affect public life, then the public should be able to know how those algorithmic systems work and to make them accountable: we should be able to audit those algorithms.
Under these circumstances, algorithmic audits are a necessary way to make this technology more explainable, transparent, predictable and controllable by citizens, public institutions and also companies, either before the development of the system, during its development or a posteriori. These audits also contribute to improving the mechanisms of attribution of responsibility and accountability of algorithmic systems.
Algorithms, especially those incorporating machine learning techniques, can handle and process massive amounts of data, including personal and sensitive data. However, as has been repeatedly pointed out, algorithms are often particularly complex and opaque in their design and behavior, making it difficult to know and control how such data is processed. At the same time, it has been shown that extensive data analysis can reveal information of a sensitive nature, which the data would not show in isolation. In addition, the purpose and usefulness of these systems is not always communicated in a clear and transparent way, even as algorithms are increasingly implemented to replace tasks previously performed by humans, including organization, prediction, recommendation, or decision-making support, among others.
Eticas Consulting (2021). Guide to Algorithmic Auditing.
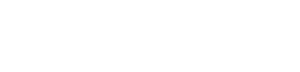
If you are using or intending to deploy a new algorithm, or if you are concerned about a third-party algorithm, Eticas Consulting can help you. At Eticas, we are experts in the development of Algorithmic Impact Assessments, which can minimise the negative social impacts of algorithmic systems and identify and the main sources of bias, both internally –if you own the algorithm– and externally –if you are concerned about a third-party algorithm–.
By auditing an algorithmic system, we’ll be able to establish its social desirability, which will depend on its capacity to follow the legal requirements in a specific context –data protection and privacy– and to anticipate and mitigate undesired social impacts while complying with the purpose that it was designed for.
Auditing algorithms can also prevent biases, which are an iterative risk that encompasses all algorithmic life-cycle: that’s why it is important to be aware of that risk at every stage of the whole algorithmic process.
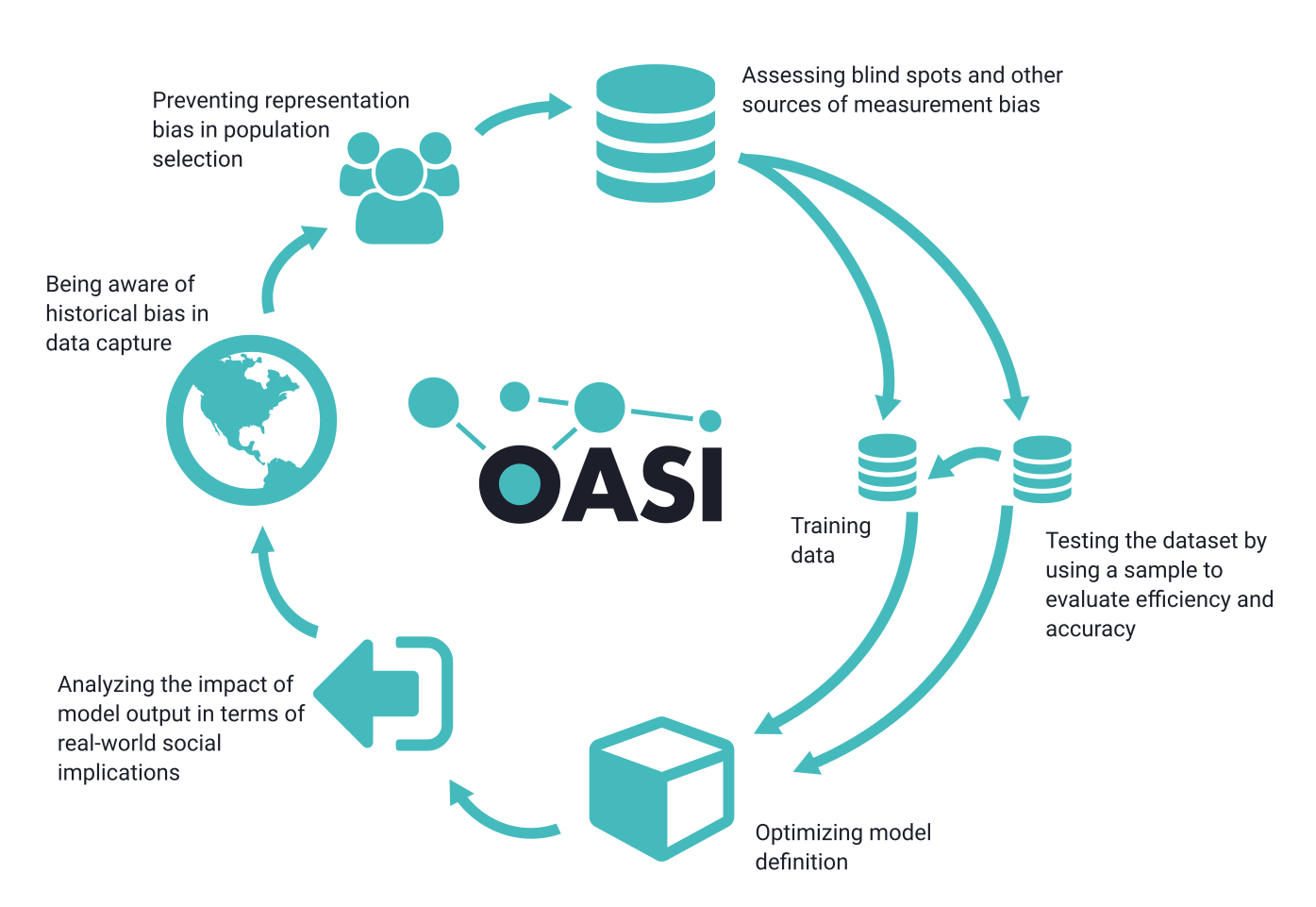
Interested in our work?
You can collaborate with the project by sharing with us algorithms that are being implemented around you or by using the information in this directory to foster changes in your community.