Community-led AI Audits:
Methodology for Placing Communities at the Center of AI Accountability
This article is based on a paper presented at the Participatory AI Governance Symposium, where we discussed the methodology and impact of Community-Led Audits.
The symposium, an official side event of the AI Action Summit, brought together experts, policymakers, and community leaders to explore innovative governance models for AI accountability.
Our paper highlights real-world case studies, methodological insights, and the transformative potential of participatory audits in reshaping AI oversight.
The Problem:
AI’s Hidden Harms
AI systems influence hiring, social services, and law enforcement, often reinforcing discrimination. Without meaningful input from affected communities, traditional audits fail to capture real-world harms.
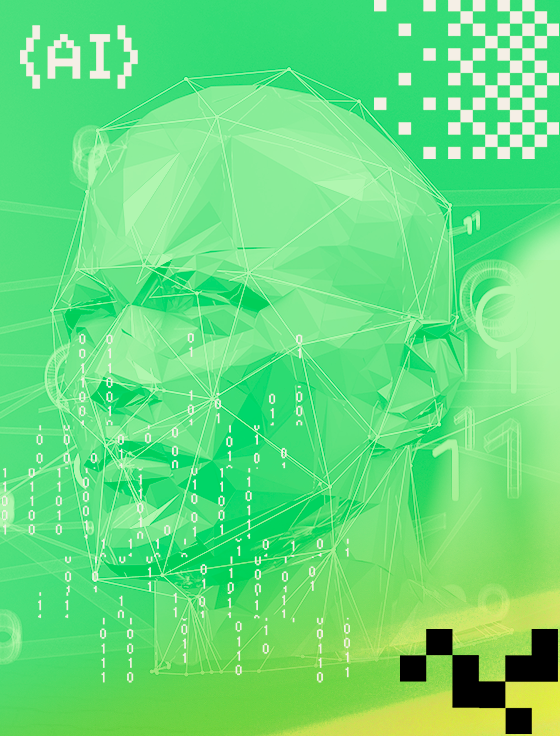
The Solution: Community-Led Audits
Traditional audits miss critical lived experiences. CLAs empower communities, expose biases, and drive change—ensuring AI serves all, not just developers. By combining technical expertise with community knowledge, CLAs provide a more complete and actionable picture of algorithmic impact.
AI accountability starts with community involvement. CLAs are a powerful tool to challenge AI harms, push for transparency, and create fairer systems. With AI’s growing influence, participatory audits are more crucial than ever. It’s time to embrace community-led auditing for a just AI future.
Eticas’ CLAs ensure affected individuals actively participate in AI audits:
01
The first step in a CLA is determining which AI-driven system is causing harm or concern within a community. This process is highly participatory, as community members—who directly experience the impact of these systems—play a crucial role in identifying AI applications that require scrutiny. For example, community members might report discriminatory outcomes in hiring algorithms, biased policing through predictive crime tools, or unfair loan rejections due to AI-based credit scoring. By focusing on lived experiences, CLAs prioritize audits of systems with real and significant impacts.
02
Once a system is identified, auditors work with the community to develop a deeper understanding of its operation, effects, and stakeholders. This stage involves extensive research, including:
- Interviews and focus groups with affected individuals to document personal experiences with the AI system.
- Technical analysis to uncover how the algorithm processes inputs and generates outputs.
- Stakeholder mapping to identify the developers, decision-makers, and entities responsible for deploying the AI.
- Regulatory review to assess legal frameworks governing the AI system.
This combination of qualitative and quantitative research ensures that the audit reflects both the systemic and human-level impacts of AI deployment.
03
A crucial component of CLAs is participatory data collection, where communities actively contribute to gathering evidence about the AI system’s operation. Different methodologies are used depending on the accessibility of the AI system:
- Crowdsourced audits: Community members voluntarily submit data about their interactions with the system.
- Experimental testing: Researchers and participants design experiments to test biases within the AI system.
- Ethnographic studies: Long-term engagement with affected communities helps document systemic trends and patterns.
- Scraping and open-source investigation: Where possible, publicly available data is collected and analyzed to identify biases or unfair practices.
By engaging communities in data collection, CLAs ensure that audits are informed by first-hand experiences rather than solely by external technical evaluations.
04
Once data is collected, it is analyzed using both technical and community-driven insights. Findings are then translated into concrete action, including:
- Advocacy campaigns: Public awareness efforts highlighting AI harms and calling for accountability.
- Policy recommendations: Proposals to regulators and lawmakers for improved oversight and AI governance.
- Legal action: Where necessary, findings may support lawsuits or policy interventions against discriminatory AI practices.
- Community empowerment: Educating affected groups about their rights and how to challenge unfair AI decisions.
By centering the voices of those most impacted, CLAs turn algorithmic audits into powerful tools for systemic change.