BadData: The High Cost of Poor Data Quality
Ignoring data quality has risks and consequences, and it can lead to costly errors, lost revenue, and damaged reputation. We explore the impact of BadData on business operations, decision-making, and customer trust.
88%
companies that reported some form of data inaccuracy
€13.5M
cost of BadData per year for an organization
€3.5M
average cost of a data breach in 2020
When big data goes wrong, that is Bad Data. From inconvenient marketing calls that got your phone number from third party companies you gave your data to without reading the terms & conditions, to more serious issues like racial profiling or credit card fraud, Bad Data affects all of us one way or another.
The effects of BadData can be mitigated through measures such as data validation, quality control, and ethical practices. However, addressing BadData requires a concerted effort from all stakeholders involved in the data lifecycle, including data producers, consumers, and regulators.
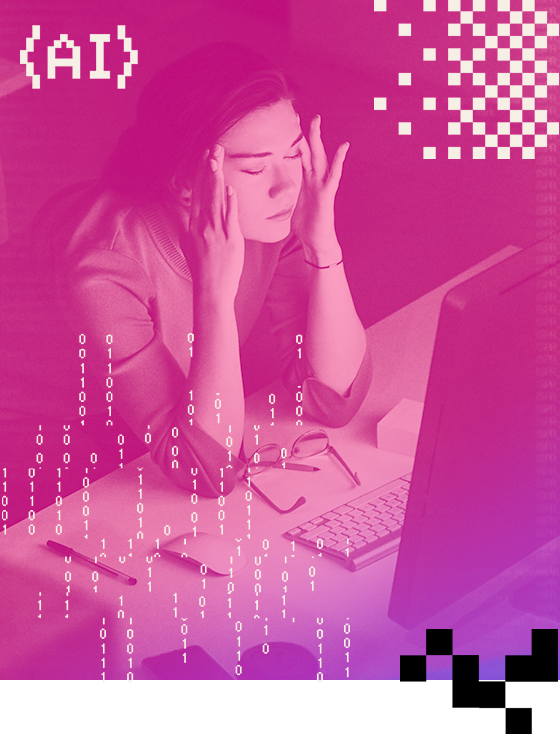
BadData is any data that is incorrect, incomplete, inconsistent, outdated, or irrelevant, which can lead to poor decision-making and other negative consequences. It can arise from a variety of sources, including human error, system glitches, and intentional manipulation.
BadData is any data that is inaccurate, incomplete, outdated, or irrelevant, which can lead to poor decision-making and other negative consequences. It can arise from a variety of sources, including human error, system glitches, and intentional manipulation. Data governance and management practices are key to ensure data quality.
To explore its effects on our everyday lives, we conducted a survey about people’s experiences with BadData. Through our research, we observed that from our BadData categorization, inaccurate data was the most common issue reported, with many individuals having to deal with mistakes with ID numbers, data of birth, and wrong surnames. The public sector and phone companies were the sectors where most BadData stories were reported, followed by healthcare, bills/tickets, and telecommunications.
01
The problem of BadData refers to inaccuracies, biases, and errors in data that can have serious consequences for individuals and society. BadData can lead to issues such as racial profiling and credit card fraud, and affects decision-making processes that impact our daily lives. There is a need for greater data literacy among the general population and more accountability and transparency in data collection and algorithmic decision-making systems. From Eticas, we launched the BadData Challenge and campaign to raise awareness and promote data equality.
02
Eticas started the #BadDataChallenge in 2018 to collect stories of people who have suffered from the consequences of poor handling of their personal data. The objective was to raise awareness of the problem and question the idea that data systems are superior to human decisions. The campaign was successful in producing a taxonomy of BadData, but we did not receive as many stories as we had hoped for. In 2022, we relaunched the campaign using social media and collaborations with like-minded organizations to spread the campaign. During the three months, we received about a hundred stories, showing the widespread impact of BadData on people's lives.
03
In 2022, our team was able to communicate and provide practical examples of BadData in a more accessible way based on pre-existing research and categorization. The majority of the BadData stories are related to the public sector and phone companies.
At least 42 stories respond to the issue that personal data was not introduced and processed accurately. Inaccurate data in public administration usually leads to time consuming and bureaucratic experiences at best, but can also end up in more complex and serious situations related to identification, payment issues, or even health problems.
These experiences are alarming and could endanger the health and well-being of a person. Within the phone and telecommunication sectors, there are many stories where data is often being illegally leaked to other services for commercial purposes.
04
- Standardize data collection: ensuring data is collected in a consistent manner. This can include implementing data collection protocols, providing training for staff, and using standardized data collection tools.
- Conduct regular data quality checks: including checking for missing data, inconsistencies, and errors in data entry.
- Implement data governance policies: this can include policies around data access, storage, and sharing.
- Use data cleaning tools: tools that identify duplicates, correct spelling errors, and remove outliers.
- Invest in data analytics: data analytics can help identify patterns and trends in data. Investing in data analytics tools and personnel can help an organization stay on top of potential data quality issues.
- Encourage a culture of data quality: providing training for staff, recognizing and rewarding good data quality practices, and emphasizing the importance of data quality in decision-making.
05
BadData is a significant problem that needs to be addressed. It is crucial that individuals and organizations take proactive steps to ensure the accuracy and integrity of their data.